The Multi-Touch Attribution Problem: Is it Still Relevant?
- Kasia Wozniak
- Aug 6, 2024
- 4 min read
In today's complex digital marketing landscape, understanding how different channels work together to drive customer conversions is key. Multi-Touch Attribution (MTA) has been the go-to tool for this, but it's facing increasing challenges and common misunderstandings. Let's explore why and discuss some more effective alternatives.
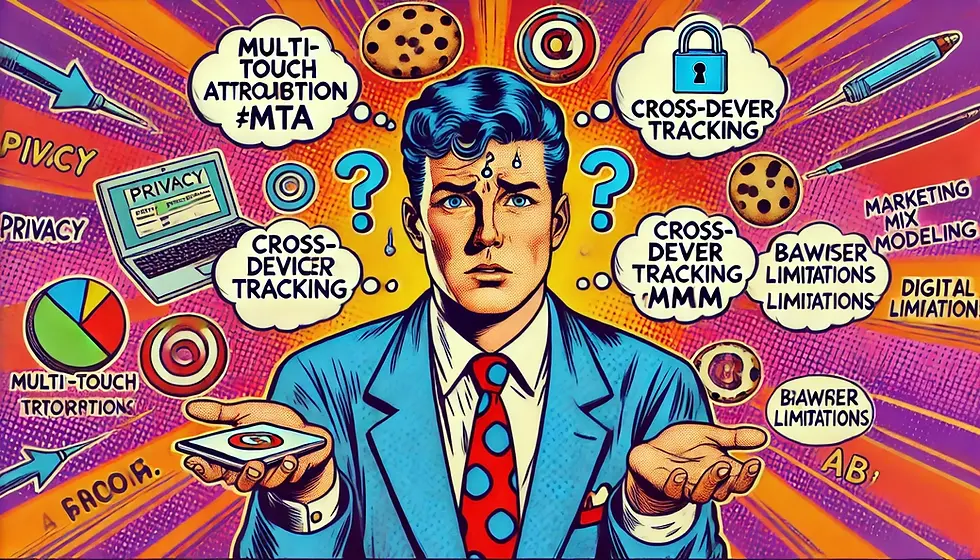
The MTA Illusion: The Cracks are Showing
MTA aims to track every step a customer takes before making a purchase, giving credit to each interaction along the way. In theory, it sounds great, but in practice, it's not always so simple.
Privacy Matters: Stricter data privacy laws like GDPR and CCPA, alongside increasing use of privacy tools such as ad blockers and incognito browsing, mean MTA can't track everything. It's like trying to put together a puzzle with missing pieces.
Cross-Device Tracking Issues: With users frequently switching between devices (e.g., from a smartphone to a laptop), MTA struggles to accurately track these interactions. This results in fragmented data that doesn't reflect the true customer journey.
Browser Limitations: Different browsers, especially those prioritizing privacy like Safari, limit cookie tracking and other data collection methods. This makes it difficult for MTA to follow users across their web activities.
The Offline Disconnect: Even online-only businesses get sales from word-of-mouth. MTA usually misses these offline touchpoints, creating a blind spot in your data.
Too Many Choices: Google Analytics offers a bunch of different MTA models, each with its own way of assigning credit. This leaves marketers confused about which model is the "right" one.
Inaccurate Estimations Due to Data Gaps: While there are various statistical methods to calculate MTA without relying solely on Google Analytics, data gaps related to cross-device tracking issues, browser limitations, and privacy concerns make it challenging to estimate the impact of each interaction accurately. These gaps lead to interrupted customer journey data, resulting in unreliable estimations.
Common Misunderstandings about MTA
Many marketers believe that MTA can perfectly track every customer interaction across all devices and touchpoints. However, due to privacy regulations, cross-device tracking challenges, and browser limitations, MTA often struggles to track every interaction accurately. This can lead to incomplete data and flawed attribution models.
Some marketers think MTA is synonymous with last-click attribution, where the final touchpoint before conversion gets all the credit. MTA aims to distribute credit across multiple touchpoints, but many marketers still rely on or default to last-click models because they are simpler to implement and understand.
Marketers might assume MTA can incorporate all relevant customer interactions, including offline and non-digital touchpoints. In reality, MTA primarily focuses on digital interactions and often misses offline activities like in-store visits, phone calls, or word-of-mouth referrals.
MTA often emphasizes direct, short-term interactions and conversions, leading marketers to undervalue the long-term impact of brand awareness and other indirect influences. Brand-building activities and long-term customer relationships often play a crucial role in conversions but are challenging to measure accurately with MTA.
As privacy becomes a bigger deal and technology changes, MTA's limitations and misunderstandings are only going to get worse. It's time to look for better solutions.
Marketing Mix Modeling (MMM): The Big Picture
In contrast, Marketing Mix Modeling (MMM) is a method used to measure the overall impact of marketing activities on business outcomes. MMM analyzes the aggregate effects of various marketing efforts, both online and offline, and takes into account external factors such as economic conditions, seasonality, and competitive actions.
MMM uses historical data and statistical analysis to determine how different marketing channels contribute to overall business performance. It provides a holistic view of marketing effectiveness, helping businesses make strategic decisions about budget allocation and campaign planning.
While MMM isn't a quick fix and requires a lot of data and statistical know-how, it offers a strategic view that MTA can't, helping you make significant decisions about your marketing budget and where to focus your efforts.
Example: Understanding Facebook Ad Campaign Outcomes
MTA Perspective:
Suppose your company runs a Facebook ad campaign. Using MTA, you might find that 20% of your sales are directly attributed to users who clicked on the Facebook ad. This is because MTA often uses last-click attribution, which gives credit to the final interaction before the sale. For example, if a customer clicks on a Facebook ad and then makes a purchase, MTA attributes that sale to the Facebook ad. While MTA can use other models like first-click, linear, or time-decay attribution to try and credit earlier interactions, these models still face challenges due to data tracking issues. Therefore, MTA might overlook significant interactions the customer had with your brand beforehand, leading to inaccurate estimations.
MMM Perspective:
In contrast, MMM takes a broader view. It analyzes the overall contribution of your Facebook ads to your sales by considering all marketing efforts and external factors. MMM might reveal that Facebook ads are responsible for a 30% increase in overall sales, capturing not just the direct clicks but also the indirect impact on brand awareness and customer consideration. MMM does this by analyzing aggregated data over time, identifying patterns and correlations between marketing activities and sales outcomes. Even if users didn't click directly from the ad to purchase, the ads influenced their decision over time by keeping your brand top of mind. This approach helps to understand the holistic impact of marketing activities without needing precise tracking of individual user paths.
Test & Learn: The Simple but Effective Approach
Another option is the "Test and Learn" approach. This involves running experiments to see what actually works. You can A/B test different ads or turn campaigns on and off to observe their impact on sales.
While A/B testing won't give you precise incremental sales figures for different marketing activities, it is an effective method to determine the most impactful way to connect with customers. By comparing different options, you can identify which strategies resonate best with your audience, thereby making your marketing efforts more effective.
Conclusion
Given the current data tracking limitations, MTA is becoming increasingly impractical. Issues like cross-device tracking, browser limitations, and offline interactions further complicate its effectiveness. While various statistical methods exist to calculate MTA, these data gaps result in unreliable estimations. Marketers need to look beyond attribution models and embrace alternative approaches. MMM and a Test & Learn approach offer more comprehensive and actionable insights into marketing effectiveness. These methods can help businesses understand what tactics work best to convert customers and make more informed, data-driven decisions.
コメント